Generative vs. Narrow AI
ChatGPT has created a frenzied interest in AI. GPT stands for “Generative Pre-trained Transformer” and is a key innovation in the course of human history. I asked Bing, Powered by ChatGPT, to explain the difference between Generative AI and Artificial Narrow Intelligence (ANI). Here is what it had to say:
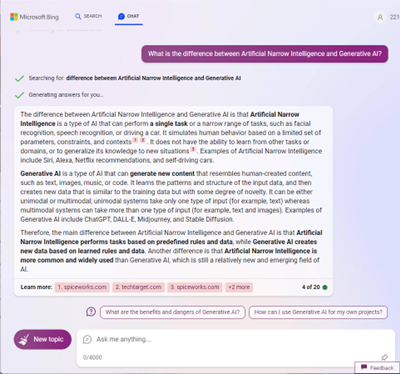
The difference between Artificial Narrow Intelligence and Generative AI is that Artificial Narrow Intelligence is a type of AI that can perform a single task or a narrow range of tasks, such as facial recognition, speech recognition, or driving a car. It simulates human behavior based on a limited set of parameters, constraints, and contexts. It does not have the ability to learn from other tasks or domains, or to generalize its knowledge to new situations. Examples of Artificial Narrow Intelligence include Siri, Alexa, Netflix recommendations, and self-driving cars.
Generative AI is a type of AI that can generate new content that resembles human-created content, such as text, images, music, or code. It learns the patterns and structure of the input data, and then creates new data that is similar to the training data but with some degree of novelty. It can be either unimodal or multimodal; unimodal systems take only one type of input (for example, text) whereas multimodal systems can take more than one type of input (for example, text and images). Examples of Generative AI include ChatGPT, DALL-E, Midjourney, and Stable Diffusion.
Therefore, the main difference between Artificial Narrow Intelligence and Generative AI is that Artificial Narrow Intelligence performs tasks based on predefined rules and data, while Generative AI creates new data based on learned rules and data. Another difference is that Artificial Narrow Intelligence is more common and widely used than Generative AI, which is still a relatively new and emerging field of AI.
The Power of Generative AI
As mentioned above, Generative AI can learn new skills and emulate human imagination. These advancements in artificial intelligence are exciting because we’re seeing for the first time, a computer able to learn abilities in a manner that is akin to human development.
The Speed and Accuracy of Narrow AI (ANI)
Artificial Narrow Intelligence (ANI), unlike Generative AI, learns its skills from humans (or maybe someday, Generative AI.) ANI models have a few benefits over Generative AI in Cybersecurity Analytics.
Terabytes and Petabytes of Signals
One thing you may have noticed in interacting with ChatGPT or other large language models (LLM) is the response to queries takes time. The question posed to Bing above took almost 30 seconds to craft. While that is a reasonable amount of time for me to wait for this answer, the rate of data signal transmission in cybersecurity analytics is in the billions/trillions per day. Because ANI is optimized and trained on a specific set of tasks and data, it can generate much faster response times.
This reduced response time is a result of lowered compute and input/output (IOPS) cycles. Lower compute cycles translate into lower costs of performing the analysis.
Data References and Validation
In the Bing response above, it cites 5 sources used in generating its response. What it doesn’t provide is the proof and validation of those sources. How did Bing determine the data presented by the 5 sources were accurate and defendable?
In developing libraries of skills and data for ANI, humans are involved in documenting what is truth and the process of validating assertions. Because these audits exist, they can be defended in internal discussions and in criminal proceedings.
Human assisted ANI provides safeguards against data poisoning and learning bad skills in a manner like a parent nurturing a child.
Cyber Logs are Digital Evidence
In my Dark Reading Article: Handcuffs over AI, I lay out the case on why cyber logs need to be treated as digital evidence. The manner that data is collected, analyzed, and preserved comes under extreme scrutiny by defense attorneys. Human experts must explain how they arrived at a conclusion and validate their credentials to the jury. The current state and nature of GPT fails to provide a definitive explanation of methods analysis.
Precinct of AI’s
To deliver a Petabyte capable Cyber Security Analytics platform, WitFoo created 5 types of ANI to perform a subset of tasks necessary to automate cybersecurity operations (SECOPS).
- Examiner – Understands how to collect, comprehend, process and preserve digital signals (messages) into forensic artifacts.
- Detective – Analyzes artifacts looking for evidence of crime that match the modus operandi (MO) of various cyber violations and criminal activity.
- Patrolman – Understands the technologies on it’s “beat” and knows how to interact to collect information or take remediation steps.
- Lieutenant – Analyzes the work of Detectives and Patrolman to generate reporting on the “Precinct” efficiency and readiness.
- Captain – Coordinates intelligence and operations with other Precincts across the CyberGrid.
This approach allows a single “Precinct” to staff up by generating and retiring the AI workers as needed. When their queues become large, more AI’s launch. When queues shrink, the AI’s are retired to reduce compute costs.
The multi-ANI approach allows for autonomous processing of data and coordination of operations without the need for human configuration.
Human Assisted ANI
As human operators interact with the data created by Narrow AI entities, the feedback is shared as interrupt to inform AI decision making. If a human flags a rogue device as a VoIP phone, the data is updated, and the AI will look for other VoIP using the same criteria.
If an Examiner AI does not know what a signal is, it is routed to WitFoo Library for Machine Learning analysis of the message and human researchers determine what the message is. The new definitions are transmitted to all Examiner AI needing the definition. This allows WitFoo R&D to deliver high-speed, accurate definitions and the Precinct user benefits with no effort.
If a Detective AI sees an attack on the network that cannot be resolved with coordination with Patrolman AI, the human is alerted for assistance. The human provides feedback to the Detective AI’s to prevent future need for human involvement.
Generative AI Assisting Humans
As Generative AI matures, the exceptions listed above can be fed to it ahead of interacting with the humans to reduce human error and response times.
Summary
Artificial Narrow Intelligence (ANI) is a powerful method for automating the skills humans possess at the rate of billions or trillions of cycles per day. ANI also accurately executes the work in a manner comprehensible to the average human. Lastly, the low compute costs of ANI make AI available to the widest possible market.
Having an army of AI that rapidly perform the cybersecurity work we know how to do, allows humans to focus on creating new skills and knowledge to teach to the Generative and Narrow AI.